In finance, extracting actionable insights from data is paramount. But to do so, we must ensure our data is correct. This is where a process called data cleaning or data cleansing comes into play.
In this blog, we get into the nuts and bolts of data cleaning techniques. We'll also provide practical data cleaning steps to help guide you through the process.
Table of contents:
- What is data cleaning?
- The importance of data cleaning
- Why manually cleaning data is so difficult
- Examples of data cleaning in finance
- Data cleaning techniques and tips
What is data cleaning?
Data cleaning is the process of identifying and correcting errors, inaccuracies, and inconsistencies in raw data. This is important because it involves removing duplicate entries, filling in missing data points, and standardizing data formats to ensure data is accurate and consistent.
Financial data must be ‘clean’ to be used for analysis and data visualization. By cleaning the data, you can prevent errors and incorrect insights, which can be a costly and time-consuming mistakes further down the line.
But what exactly is ‘clean data’?
According to TechTarget, characteristics of clean data include:
- Accuracy
- Completeness
- Consistency
- Integrity
- Timeliness
- Uniformity
- Validity
Why is data cleaning important?
Data cleaning is super important, and here's why. First off, it's all about making sure your data is accurate. Think about it - if you're working with what's known as 'dirty data', the analysis can go haywire. This can lead to misleading results, misinformed decisions, and potential financial loss.
Secondly, clean data makes the whole process of data handling and analysis run a whole lot smoother. Who wouldn't prefer working with a neat, orderly dataset over a chaotic one? It just makes everything (including data processing) more efficient.
Finally, when your data is clean, your predictive models turn out to be much more reliable. These models are kind of like picky eaters - they perform best when you feed them good, clean data. So, with your data in tip-top shape, you'll be in a better position to forecast future trends and make smart, proactive decisions.
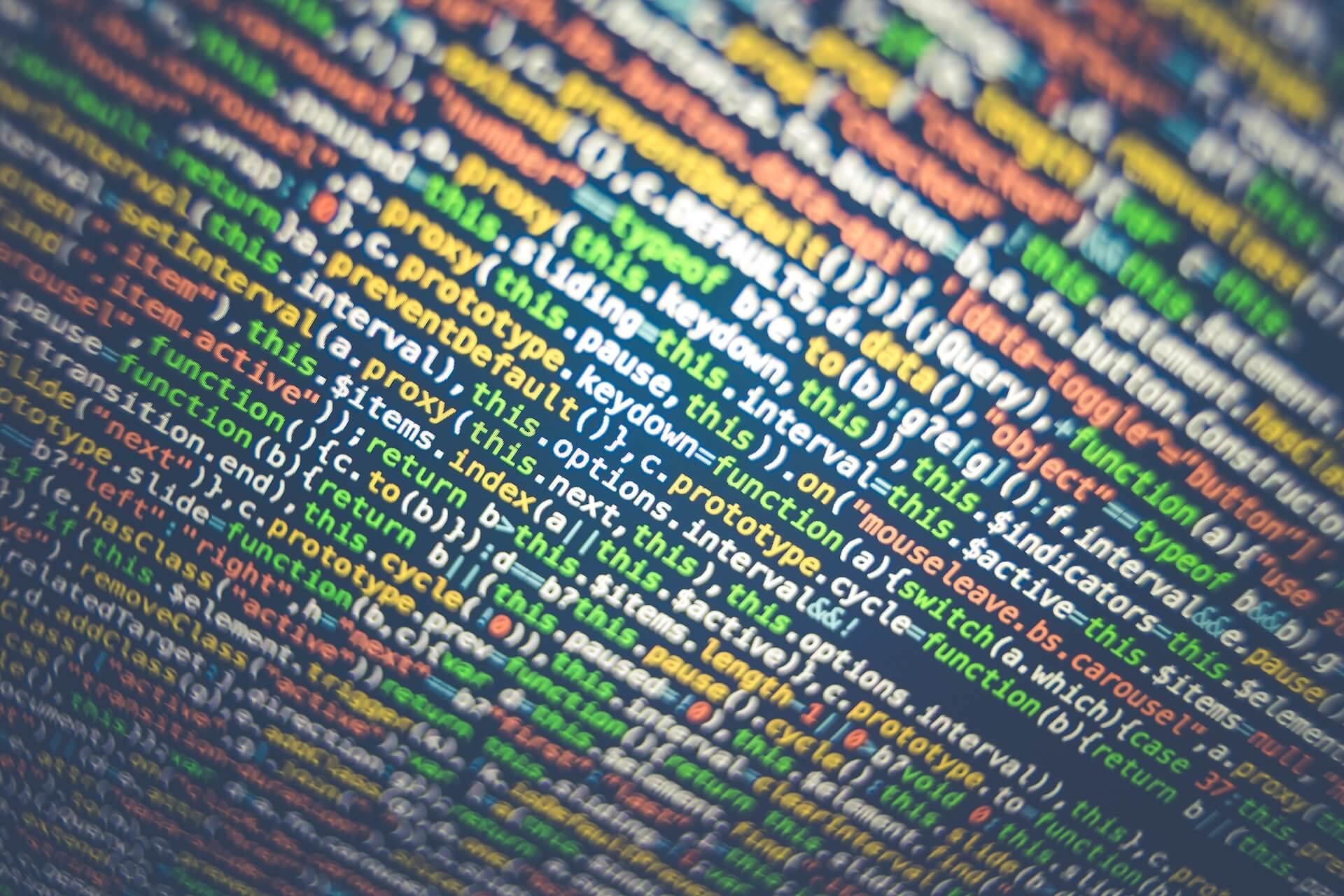
What makes manually cleaning data challenging?
One of the main challenges of manually cleaning financial data is the sheer volume of information on hand. In today's world, finance professionals manage massive datasets, which can quickly become overwhelming. After all, making sure no errors are overlooked takes time, patience, and meticulous attention to detail.
Secondly, data discrepancies can often be subtle and hard to detect. Identifying and correcting these can be a daunting task. Some examples include issues in the data, like differing data formats or misspelled words.
Lastly, there's the risk of introducing new errors during the data cleaning process. For instance, while filling in missing data or removing duplicates, one might accidentally delete critical information or input incorrect data.
Data cleaning in finance examples
Examples of data cleaning in finance can vary but here are a few common scenarios:
Duplicates in a transaction database
Let's say you're pouring over a massive list of customer transactions. As you sift through, you start noticing some suspiciously identical entries - same customer, same date, same amount, etc. That's a classic example of duplicate data. In data cleaning, your first order of business is to seek out and remove these sneaky repeats.
Inconsistent currency formats
When working with a dataset from multiple countries, you'll often work with different currencies. One dataset can include figures in US dollars, Canadian dollars, euros, etc., and analyzing mixed data like this can be difficult. You'd need to convert all those different currencies into one standardized format.
Consolidating financial statements
Suppose a CFO is overseeing multiple business units, each with its own financial statements. In this case, data cleaning might involve aligning the data structure across all these units, ensuring the same account names and financial categories are used, so that a consolidated report can be prepared accurately.
Handling different fiscal year ends
If the CFO is managing companies or subsidiaries with different fiscal year-ends, they'll need to standardize the data for comparison or consolidation. Data cleaning in this scenario involves aligning data into a uniform fiscal period.
Discrepancies in revenue recognition
In some cases, different business units might recognize revenue differently. For instance, one on a cash basis and another on an accrual basis. Data cleaning is needed to standardize the revenue recognition method across all units for accurate reporting and analysis.
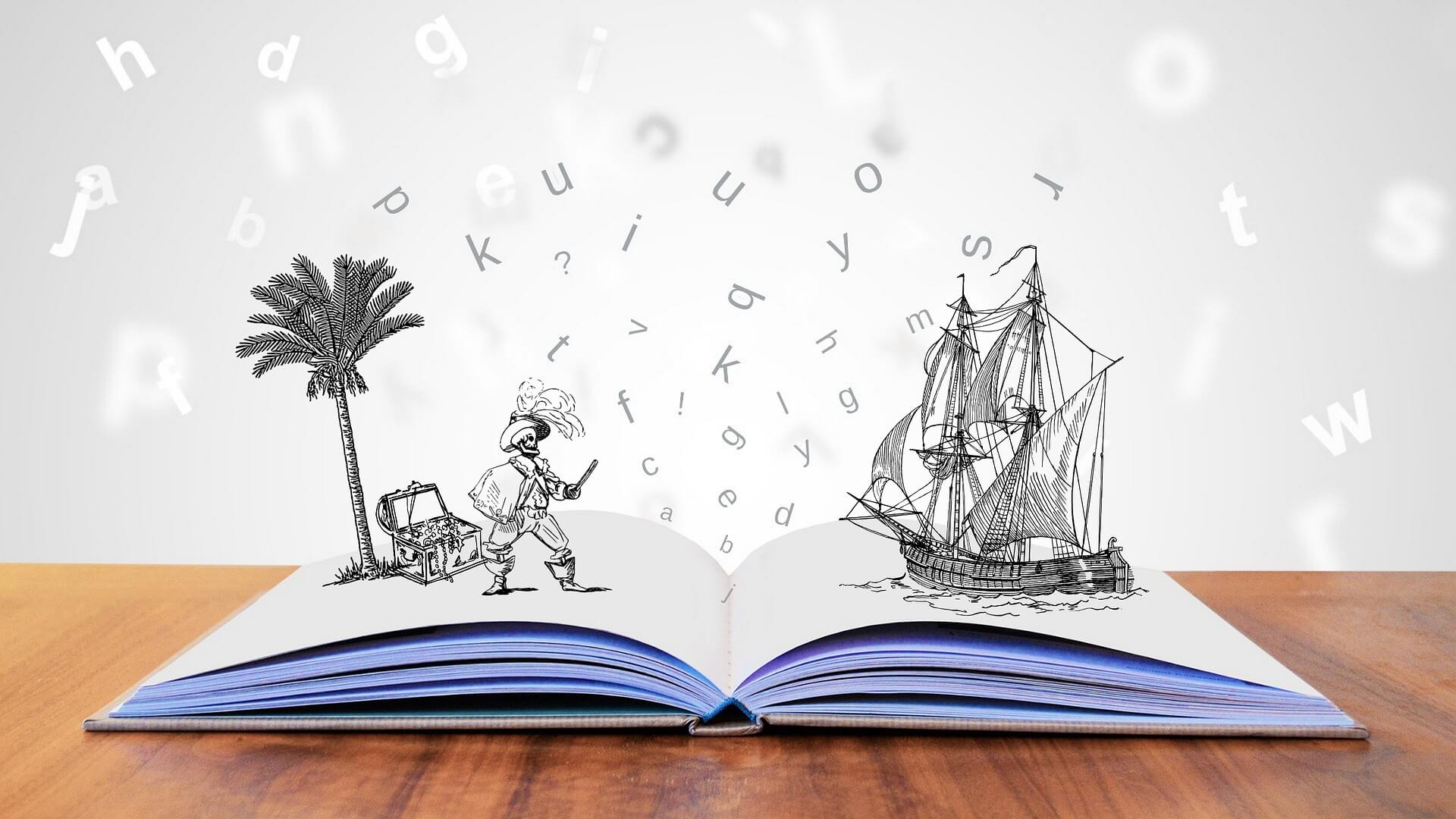
Data cleaning techniques in finance
There are a few commonly used data cleaning techniques to help ensure data is clean and free from mistakes. Here are a few:
1. Remove duplicates
Duplicated data entries are more common than you might think and tend to occur during data collection. This can lead to inconsistencies and errors in your analysis and visualizations. By removing duplicates, you can ensure your data is accurate and consistent.
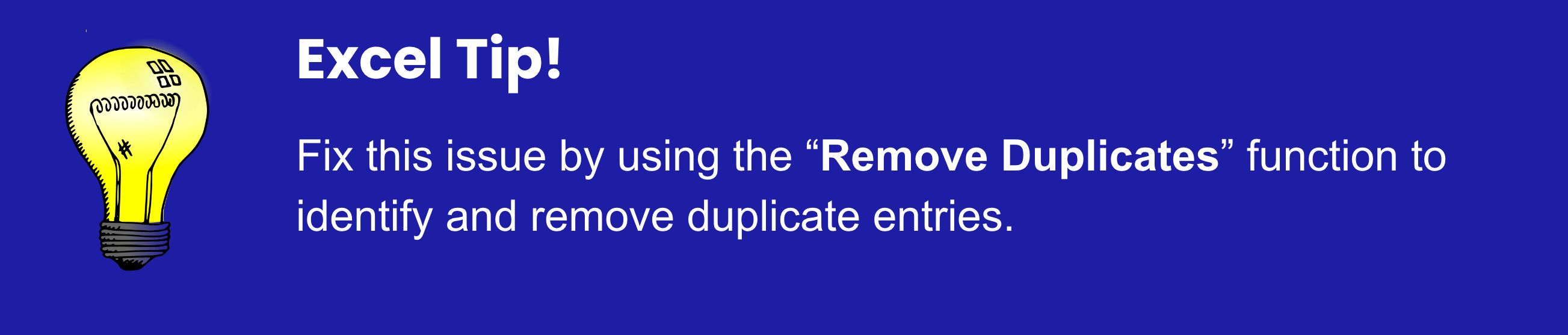
2. Fill in missing values
Have you ever tried to solve a puzzle with missing pieces? It’s frustrating! The same goes for missing data in your dataset.
Missing data can be a major problem when it comes to analysis. It can skew your results and make it difficult to draw accurate conclusions. By filling in missing values, you can ensure your analysis is based on complete and accurate data.
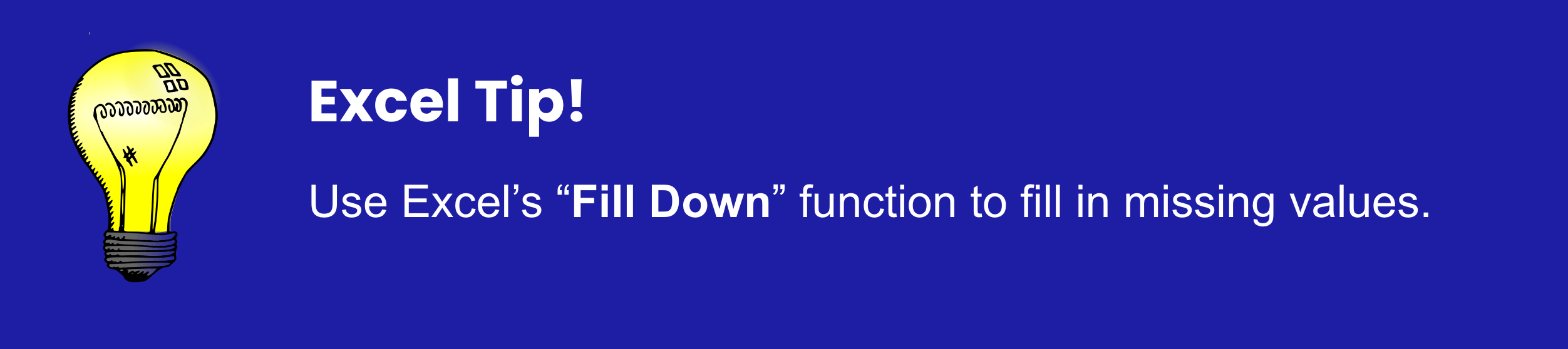
3. Correct inaccuracies
Inaccurate data can lead to incorrect insights and decisions. Taking time to correct errors ensures your data is reliable and trustworthy.
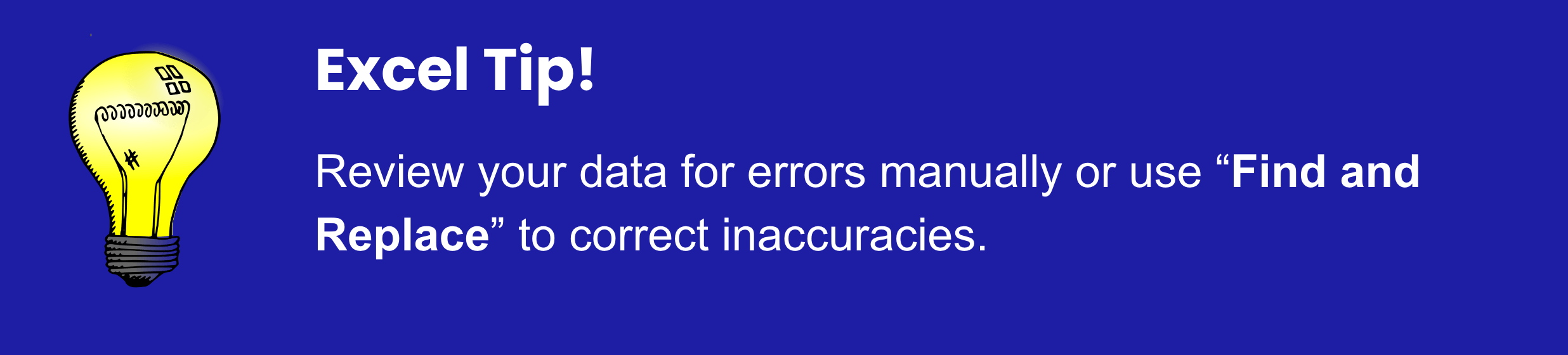
4. Standardize data formats
Standardizing data formats ensures your data is compatible and easy to work with. If your data formats are inconsistent, it can lead to more errors.
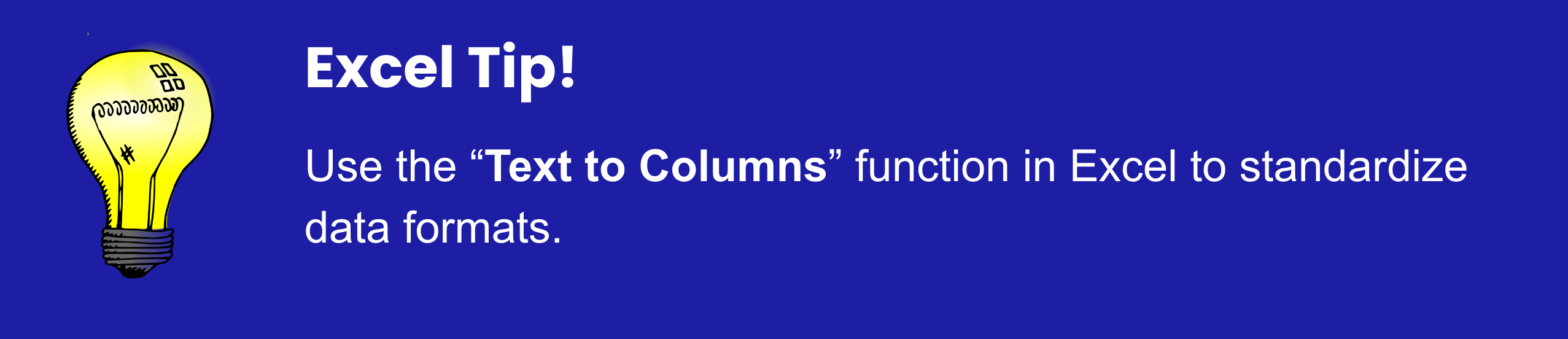
5. Remove irrelevant data
Irrelevant data can clutter your dataset and make it difficult to draw meaningful insights. Removing unnecessary data lets you focus on the most important information.
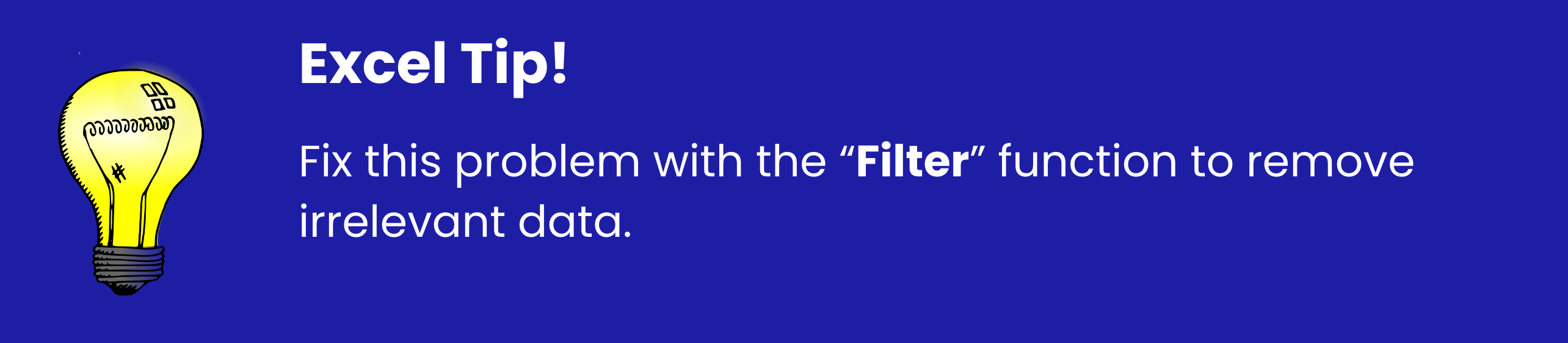
FAQs: Data cleaning
What are the 3 objectives of data cleaning?
The main objectives of data cleaning are to enhance data quality by removing errors and inconsistencies, to prepare the data for analysis and visualization and to ensure reliable and accurate decision making based on the data.
What data should be cleaned?
All data, regardless of its source, should be cleaned before analysis. This includes data from spreadsheets, databases, text files, and even data collected through forms and surveys.
Is data cleansing a part of extraction?
Yes, data cleansing can be part of the extraction process, often referred to as the ETL (Extract, Transform, Load) process. However, it is also a standalone process that needs to be performed continuously as new data is added.
Download the Storytelling with Data Visualization Playbook
Tired of presenting financial data that falls flat? Frustrated that your insights are lost in a sea of numbers and charts? Worry no more! We've created the ultimate playbook to help you transform your financial data into captivating, persuasive stories.
Discover the essential components of a powerful data narrative and learn how to weave them together to create a story that resonates with your audience.
What's Inside?
✅ A step-by-step guide to crafting compelling data stories
✅ Financial data preparation and cleaning tips
✅ Expert tips on selecting the right graphs and charts
✅ Best practices for engaging presentations that inspire action
✅ How to build a persuasive argument with financial data
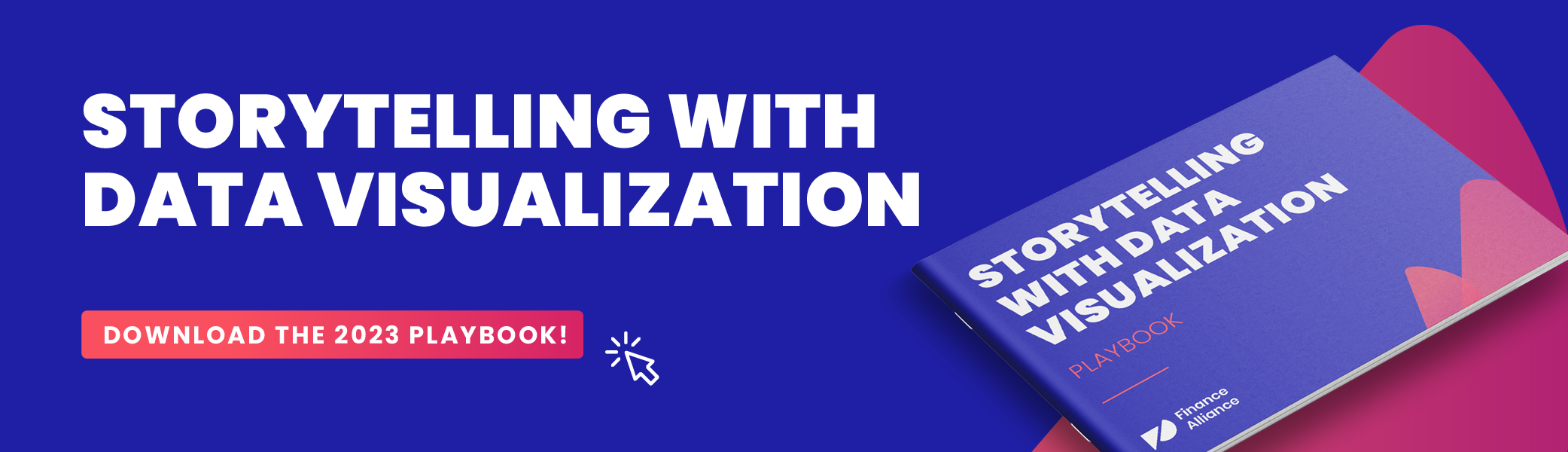