AI is emerging as a key differentiator in enterprise finance. As traditional financial models struggle to keep up with the pace of change, enterprise tech organizations are turning to AI to unlock faster, more accurate, and insight-driven decision-making.
Drawing from my experience in sales planning and forecasting in the enterprise tech sector, I’ve seen firsthand how AI is reshaping how global enterprises forecast revenue, optimize GTM strategies, and manage P&L risk.
This article explores how AI is transforming financial modeling and sales forecasting (two pillars of enterprise strategy) and helping finance teams shift from reactive to proactive operations.
1. Why traditional forecasting falls short
There are three main reasons why traditional forecasting is falling short:
Lack of broader business context
Sales forecasters and financial modelers frequently lack visibility into wider organizational shifts such as changes in product strategy, marketing campaigns, or operational execution that affect demand and performance. This makes it difficult to fine-tune models for niche business dynamics or rapidly changing market conditions.
Inflexibility
They often have an inability to account for real-time changes in demand, market shifts, economic conditions, tariffs, or sales performance.
Human bias
Over reliance on gut-feel projections leads to inaccurate financial planning.
In many enterprise settings, these limitations create friction between planning and execution across business functions, finance, sales, and marketing. Misaligned forecasts result in delayed strategic actions and misused resources, which are issues that AI is now well-positioned to solve.
2. What makes AI a game-changer for financial modeling
Cross-functional simulations tailored by domain experts
One of AI’s most transformative strengths lies in its ability to empower every function within the enterprise to personalize simulations using their domain-specific expertise. For example:
- The pricing team can continuously adjust models based on real-time strategy updates.
- The product team can simulate outcomes tied to roadmap changes or launch timing.
- The marketing team can incorporate variable lead generation budgets or campaign performance assumptions.
Likewise, GTM leaders can simulate how scaling inside sales headcount could drive more transactional business and enhance margins. These deeply integrated, cross-functional simulations not only improve forecast precision but also drive strategic alignment and execution agility across the business.
Real-time forecast adjustments
Unlike static quarterly models, AI allows finance leaders to refresh forecasts dynamically, giving real-time visibility into revenue performance. This is particularly useful in fast-evolving segments like AI infrastructure, where product cycles and demand signals change rapidly.
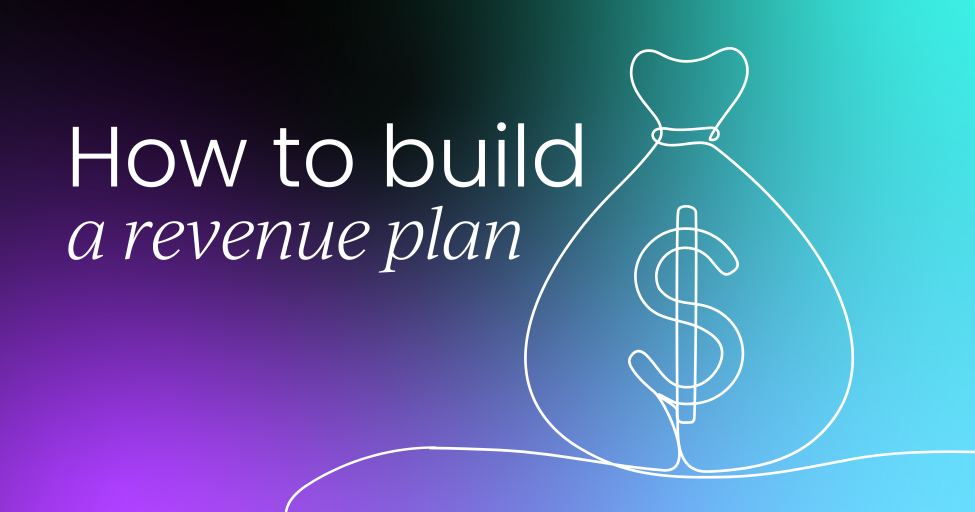
3. Practical use cases in enterprise finance
AI-powered lead scoring & targeting
Inspired by the Lean Startup's 'Build-Measure-Learn' cycle, one effective AI use case is building a lean, predictive lead scoring model.
Organizations can:
- Develop an initial AI model based on historical data to identify high-probability buyers.
- Continuously refine lead targeting with real-time behavioral and market data.
- Deploy a pilot program with a focused sales team to test and validate the model’s effectiveness.
- Measure conversion rates, learn from outcomes, and iterate the scoring logic.
Smart bundling & pricing optimization
Following lead scoring, enterprises can create value by applying AI to product bundling and pricing strategies. This includes:
- Building AI-driven recommendations for optimal hardware/software bundles based on customer profiles.
- Integrating dynamic pricing capabilities that react to competitor behavior and market demand.
- Running A/B pricing tests within specific customer segments to evaluate effectiveness.
- Collecting feedback from sales teams to iteratively enhance pricing logic and usability.
Automated revenue forecasting
Another valuable use case involves enhancing revenue visibility and predictability. Organizations can:
- Better predict conversion rates for large strategic deals and transactional segments, enabling more reliable revenue planning across deal sizes.
- Forecast transactional business growth patterns tied to seasonal cycles, marketing triggers, or high-velocity sales channels.
- Continuously refine revenue projections by integrating demand signals, channel performance, and seasonality.
- Establish feedback loops between finance and GTM teams to adjust models based on real-world performance.
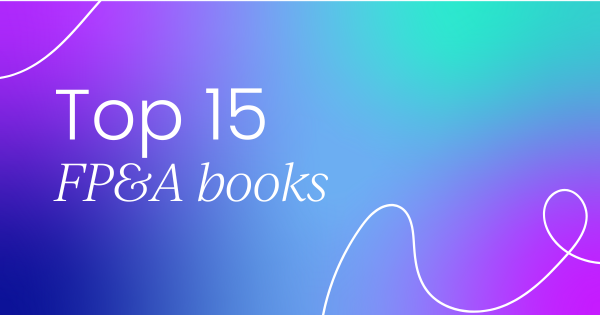
4. How AI enhances execution and GTM strategy
Smarter pipeline management
AI can streamline pipeline visibility and improve forecast reliability through:
- Collaborative pipeline reviews with finance and sales using AI-generated risk scores and close probabilities.
- Analysis of competitor dynamics and market share shifts at the product and geo level to understand how winning or losing specific deals affects strategic positioning.
- Enhanced understanding of how pipeline outcomes impact both profitability and long-term growth trajectories.
Improved sales productivity
AI boosts front-line efficiency by guiding sales teams to focus efforts on the right product segments expected to experience a surge in demand (such as those driven by OS refresh cycles, compliance deadlines, or emerging industry triggers), enabling them to strategically capture growth opportunities.
AI also helps to prioritize accounts while providing accurate bundling suggestions based on buyer profiles and sales history to increase deal size and win rates.
Tighter finance-sales alignment
AI serves as a bridge between strategic planning and operational execution by:
- Providing shared insights to drive collaboration between FP&A, GTM, and sales teams.
- Enabling joint decision-making based on real-time financial and sales data.
- Improving coordination between business units through unified performance metrics.
- Reducing misalignment and strategic blind spots across planning cycles.
5. Key considerations for implementation
- Data readiness: Clean, structured data is critical. Integrating CRM, ERP, and planning systems improves AI effectiveness.
- Human oversight: AI augments, not replaces, finance leadership. Human intuition is still key for context and judgment.
- Change management: Teams need training and adoption support to fully leverage AI’s potential.
Conclusion
AI is redefining how enterprise tech companies forecast, plan, and execute. From lead targeting to revenue modeling and cross-functional scenario planning, it brings precision, agility, and alignment to financial operations.
By breaking silos and enabling real-time collaboration across finance, GTM, and sales, AI turns forecasting into a growth engine. Companies that embed AI into their processes will be better positioned to anticipate market shifts, improve profitability, and lead with confidence.
*Disclaimer: The views expressed in this article are my own and do not reflect the official policy or position of any organization. *