My last blog about the FP&A Chasm generated a lot of buzz, so I thought I'd write a short playbook on another hot topic - "Data-Driven Decision Making" or DDDM.
Topics covered in this article:
- The Basics: Recognizing that you have a problem
- Predictive analytics
- 1-10-100 Rule
- How to avoid analysis paralysis
The Basics: Recognizing that you have a problem is the first step to solving it

The overwhelming majority of executives plot their organizations as either data-informed or data-driven on the spectrum above. However, most of them have data collection practices more characteristic of a 'data indifferent' organization. We all know the saying, "garbage in, garbage out" when it comes to analysis, so it stands to reason that you can’t be data-driven or data-informed if you don’t collect the data. At least not in the way you think.
It's common for the CRO of an organization to puff up their chest and tell me about all the salesforce dashboards they use to drive decisions. They quickly deflate when I ask them about the integrity of their salesforce data. If this is you, that’s fine! Just understand that you are not truly analyzing your pipeline, customer traction, GTM effectiveness, or your sales process.
The decisions that you are making are based on what your sales reps decided to show you. This doesn’t mean that you can’t glean insights, it just means that you have to be realistic about what data you’re actually analyzing. Are you analyzing the effectiveness of your sales process, or are you analyzing the behavior of reps? That matters.
When we analyze this type of sales-rep-biased pipeline data, even the worst data, we’ve come up with an insight that either made or saved the company $500k or more every single time.
Predictive analytics
Every organization we work with wants to move into predictive analytics (why else would they buy our platform?). If you haven’t seen this chart by Gartner covering the analytic value escalator, then you should look at it very carefully, close your eyes, and burn that image into your brain.

The famous quote by philosopher George Santayana, "Those who do not learn history are doomed to repeat it.” This is an escalator for a reason. You can never get to predictive or prescriptive analytics without tackling descriptive and diagnostic analytics first. It may seem counterintuitive, but nail the descriptive and diagnostic phase of your business and you’ll get predictive answers faster.
The human brain is the best analytical engine we have. Feed this analytical engine accurate historical analytics and you will instantly connect dots that would take analysts years to do with a mediocre data set. Focus your Organization's efforts on understanding your past, and the people at your company will be able to predict the future 10x faster than if you spent the same resources on predicting the future.
Why? Because of the 1-10-100 rule.
1-10-100 Rule

There’s a concept in manufacturing called the 1-10-100 rule that describes the exponentially increasing cost of fixing an issue as it moves through the stages of the process.
The 1-10-100 rule applies directly to DDDM! A faulty data point costs your organization exponentially more to fix the more decisions you make based on it.
For instance, if you hire additional engineers based on an inaccurate revenue forecast, the cost of unwinding that decision is exponentially more expensive than generating a better revenue forecast. Look at the debacle that is JP Morgan’s acquisition of Frank, where a little more analysis would have saved them $175,000,000.
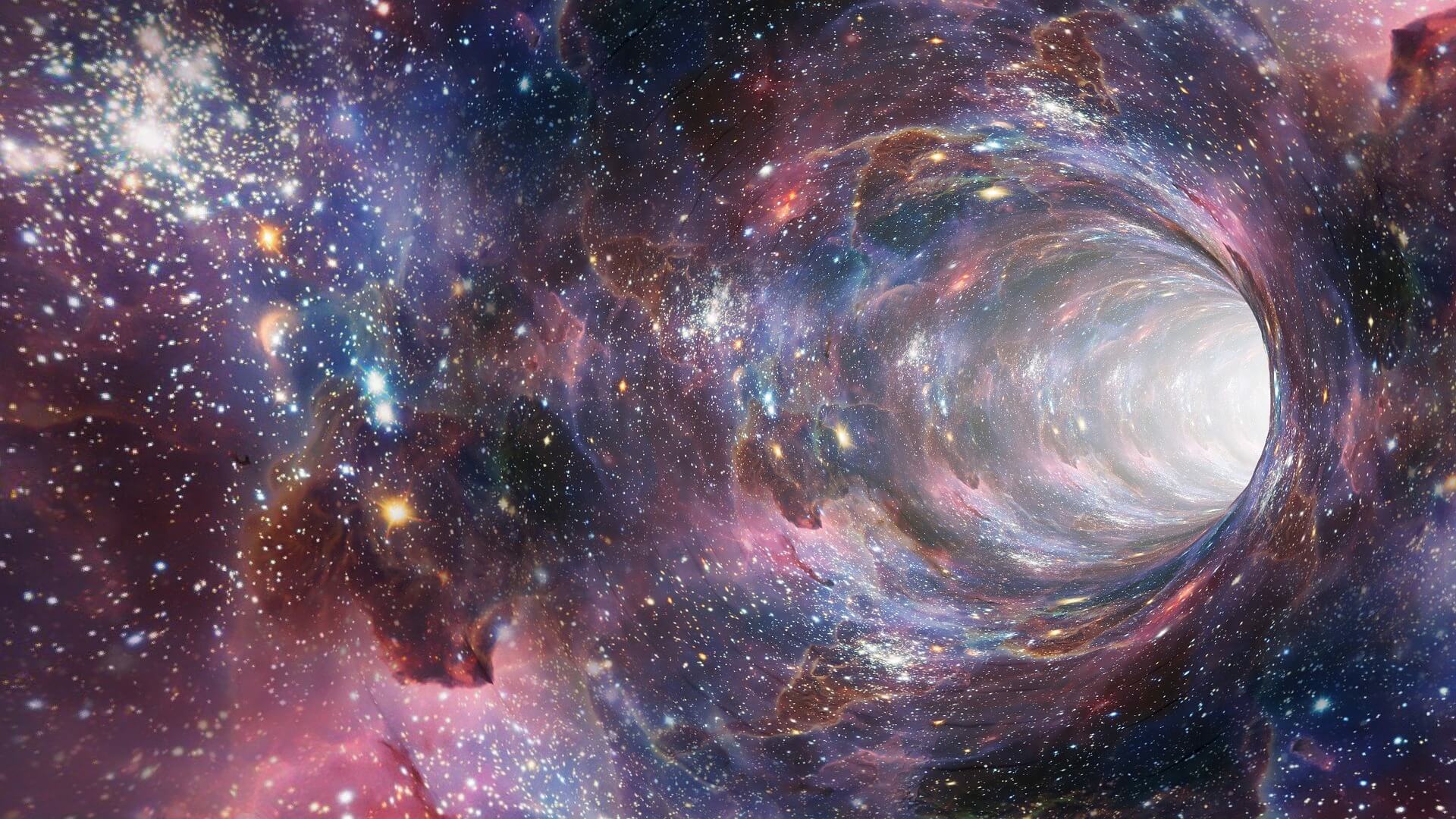
But how do we avoid Analysis Paralysis?
Use Amazon's Type 1 and Type 2 decision framework to understand when you should make a decision quickly versus taking the time to ensure you understand all the facts and circumstances.

Amazon's decision-making framework distinguishes between two types of decisions: Type 1 and Type 2.
Type 1 decisions are irreversible and can have significant long-term consequences. They are high-stakes decisions that require careful consideration and should not be made lightly. Examples of Type 1 decisions include investing in a new business line or product, entering a new market, or acquiring another company. These decisions often require a great deal of time and resources to fully evaluate all the relevant facts and circumstances. It is important to take the time to gather all the necessary information and input from relevant stakeholders before making a Type 1 decision.
Type 2 decisions, on the other hand, are reversible and have a lower level of impact. These decisions are more operational in nature and are typically made on a day-to-day basis. Examples of Type 2 decisions include changing a website layout or modifying a marketing campaign. Type 2 decisions can often be made quickly, with less input and analysis, because the potential consequences of making a wrong decision are relatively minor and can be corrected easily if needed.
By using this framework, you can determine when to make a quick decision versus when to take the time to gather all the facts and make a more informed decision. When faced with a decision, you should consider the potential impact of the decision, the reversibility of the decision, and the amount of time and resources required to fully evaluate the decision. This can help ensure that you make the right decision for your organization, based on the circumstances and stakes involved.
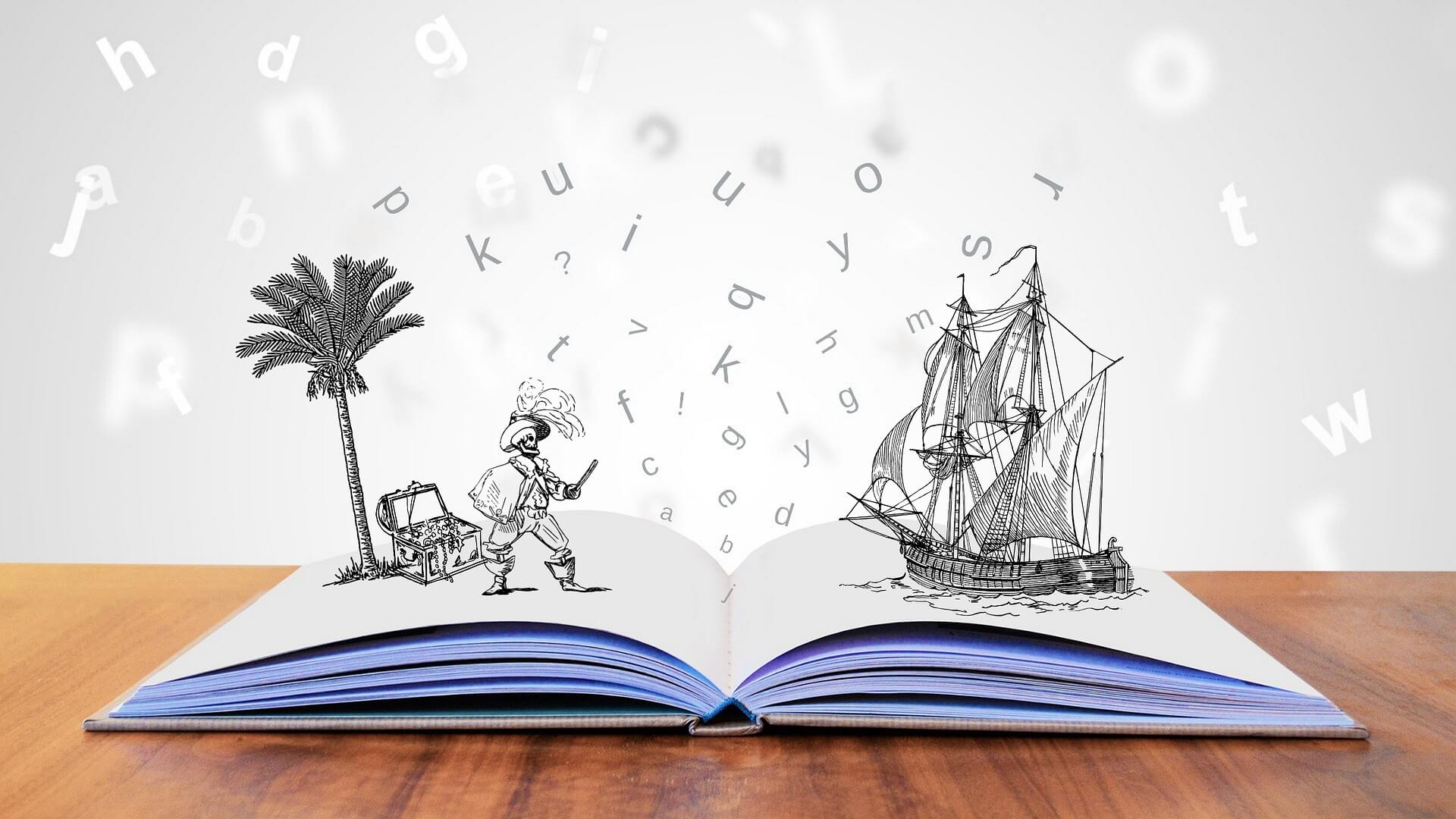
Key Takeaways
- Recognize the problem to solve it: Executives who have poor data collection practices cannot make data-driven decisions.
- Understand what you can analyze: Even the worst data is often a gold mine when you think creatively.
- Descriptive and Diagnostic Analytics are essential: You can't predict the future until you understand your past.
- The 1-10-100 rule applies to data-driven decision-making: The cost of fixing an issue increases exponentially the more decisions you make based on a faulty data point.
- Use Amazon's Type 1 and Type 2 decision framework to understand when to make quick decisions and when to take the time to gather all the facts.